What do AI alignment fears reveal about market capitalism?
There are high-level similarities between the types of incentive problems that exist in the economy and optimization problems AI programmers face.
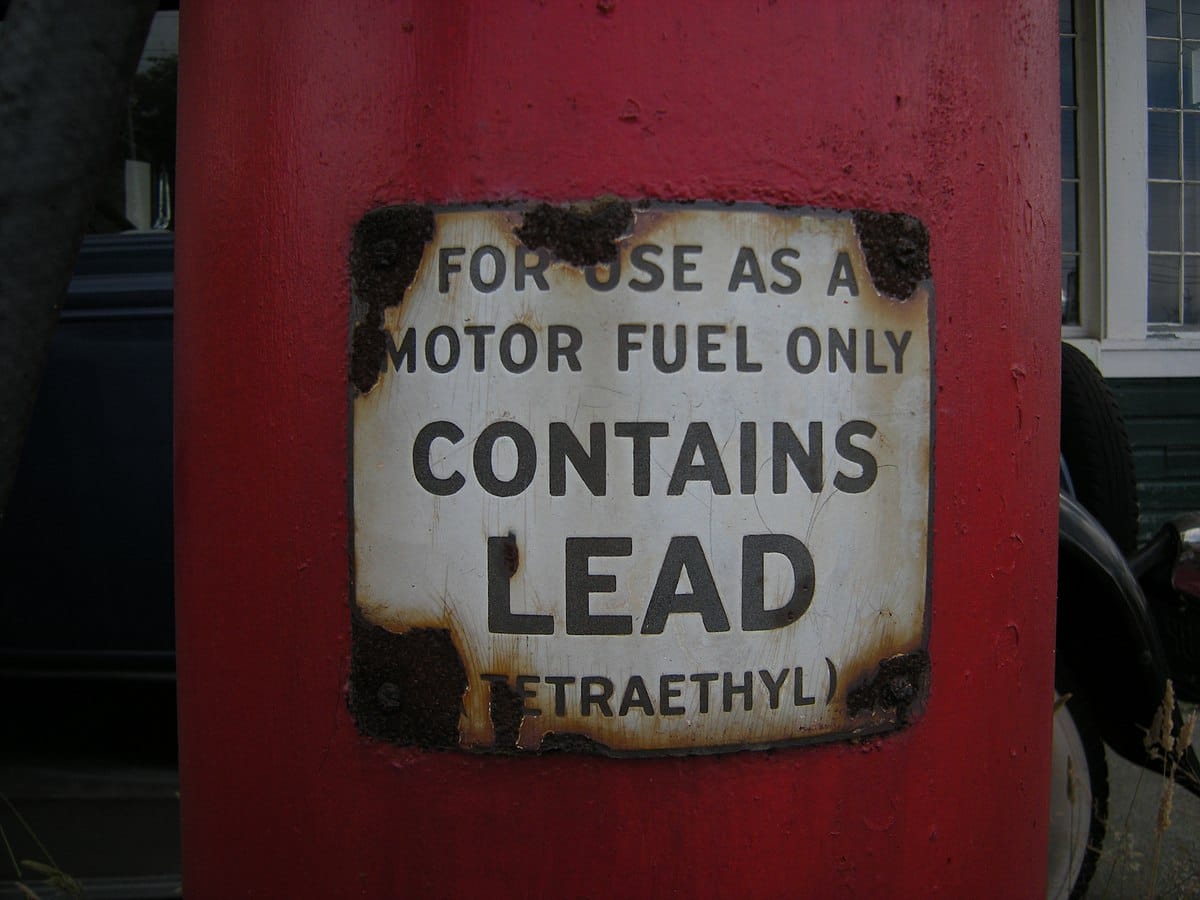
Fears of AI doom are deeply ingrained in anglophone culture, shaped by speculative fiction with disastrous endings. While this fear might seem new, it’s fairly old. As Vox’s Kelsey Piper points out, this genre of sci-fi was partly inspired by the writings of mid-20th century academics, predominantly mathematicians who worked on WWII-related technologies.
Consider John von Neumann, a scientist more prolific than Einstein, who had his hand in seemingly much of 20th-century math, information theory, and physics. Von Neumann was the first person to propose the concept of a technological singularity, a point where technology becomes so complex that it accelerates its own growth. He had also conceptualized the logical possibility of machines capable of self-reproduction. I.J. Good, another 20th-century mathematician is responsible for coining the term “intelligence explosion” in 1965. Even some ways we discuss AI performance today, like the “Turing Test” come from this generation of mathematicians and computer scientists.[1]1
For outsiders, AI literature can be difficult to navigate. Beyond the technical depth of the field, it feels like there's a tension between those who foresee runaway intelligence and those who don’t.[2]2 Often, it feels like you must pick a side before the arguments even make sense.
Does AI alignment even matter?
There’s a temptation for those of us who don’t believe in singularities, rapid recursive intelligence growth, or seemingly “omnimaximal” superintelligent AI to disregard this portion of the literature entirely. It doesn’t help that some of the public-facing support for alignment of uncontrollable machines, comes from a niche internet subculture that has spawned some of the weirdest stories in tech over the past decade.[3]3
Putting all of that aside, there are serious academics working on alignment, and the field offers a rich compilation of cybernetics, control theory, and other systems sciences.[4]4 These areas are of growing importance as the world becomes more complex and interdependent. AI alignment is relevant today not because of hypothetical superintelligence, but because it grapples with runaway optimization—a failure mode common to many technologies and social systems. In this post, we’ll explore how AI alignment failures mirror market failures and why that comparison reveals more than the Econ 101 view of market externalities.
What is AI alignment?
AI systems and the methods used to train them, can be thought of as optimization processes[5]5 with the goal of AI deployment being to solve problems on behalf of people. The alignment problem is getting these systems to pursue goals in ways that avoid unintended harms. This concern applies to systems of any scale, not just hypothetical “superintelligent AI” or ASI (artificial superintelligence). For example, programmer Brian Christian[6]6 in his book The Alignment Problem talks about instances of vision systems producing racial bias and medical inaccuracies.[7]7
Is market capitalism a misaligned AI?
AI alignment literature captures the difficulty of controlling optimization while fears of superintelligence parallel how market capitalism operates. For example, observers outside the AI alignment community have highlighted how firms and even capitalism itself resemble a dangerously unaligned AI (more so than today's AI systems, at least). See Charles Stross, Ted Chiang, Cory Doctorow, and Dominic Leggett, who each independently make this critique.
- AI “doomers” who are not explicitly AI alignment researchers, including Max Tegmark and Yoshua Bengio (one of the “godfathers of AI”) have also made similar arguments.
- Conversely, many in this AI alignment/“doomer” category reject this analogy, usually because they think it trivializes the challenge of AI alignment or because they believe corporate governance has largely solved this problem.
- AI alignment researchers like Anders Sandberg and Peter Eckersley (1978-2022) have highlighted how capitalism and crowd wisdom, more generally, resemble an intelligence with market incentives mirroring the reward functions used to build AI systems.
- Eckersley is interesting in that he argued market capitalism demonstrates gradient descent—an iterative “pathfinding” optimization algorithm used to build AI systems—and backpropagation. This would quite literally make capitalism a special kind of AI. Unfortunately, he died before fully elaborating on this provocative and extremely contentious idea.
However compelling you find the analogy, it isn’t surprising that it’s being made by so many people of different backgrounds. Market capitalism can be seen as an optimization process, with firms acting as “agents” on the public’s behalf. There are high-level similarities between the types of incentive problems that exist in the economy and problems programmers face managing algorithms.
In short, I view both AI risk and market capitalism as potential “Mammon generator” machines. Both AI and market capitalism are problem-solving optimization processes whose internal criteria for selecting solutions do not inherently avoid undesirable harms without intervention.
What does AI alignment teach us about optimization (and market capitalism)?
Today, companies are increasingly building AI systems to act on behalf of others. This could involve helping doctors analyze medical images or automating decision-making processes for expedient loan approval. For the purposes of this blog post, terms like machine learning, deep learning, AI, and agentic AI will mostly be used interchangeably.[8]8
The alignment problem occurs when a goal-directed agent—in this case, any system leveraging machine learning behaves in a way not anticipated by a developer or end user. In Brian Christian’s The Alignment Problem, Christian discusses how a dermatological imaging tool fed 130,000 images of diseased and healthy skin, began to rate images containing rulers as malignant tumors. This association was “learned” because of patterns in the training data.
While this is a simple case with a simple solution, AI designers are now building more complex systems intended to work autonomously across different domains. As this happens, diagnosing unanticipated behaviors will become more challenging. This inscrutability is central to the alignment problem. There’s a question of how we can ensure AI always does what we want, even if we’re not supervising it or able to evaluate its decisions. The issue is of growing importance as companies choose to hand over the wheel to AI, sometimes literally, as is the case with autonomous vehicles.
When thought of this way, the alignment problem is analogous to principal-agent problems in law and economics. These problems revolve around ensuring that a principal, or an individual with a key interest in some matter, can guide an agent acting on their behalf to behave in their best interests. Depending on the relationship, agents may operate with considerable leeway and sometimes greater information about conditions on the ground (a classic example of information asymmetry). We typically resolve such problems with contracts, terms and conditions, and other legal disclosures requiring both parties to “put everything on the table.” This helps increase transparency and ensure that everyone commits to only taking mutually beneficial actions for the relationship.
Programming AI isn’t contract writing, but reward functions play a similar role in guiding behavior. The alignment problem can be thought of as a programmer (the principal) needing to accurately define the reward function so that the AI (the agent) learns to identify actions that lead to desired outcomes. The programmer risks the AI pursuing unintended or harmful goals if the reward function is poorly defined.
If this sounds familiar to you, as a reader of this blog, it should. It’s Mammon! Indeed, all the problems of optimization, including overoptimization and misoptimization apply here. If the reward function is not robust enough, an AI will learn to prioritize the wrong things because the path to its goal was poorly specified. In current AI systems, this can take the form of specification gaming, where an AI can be thought of as following the letter of its given reward function, nothing more or less.[9]9
How AI Learns to Cheat | The Challenge of Specification Gaming - Jordan Harrod
Specification gaming can occur in AIs operating in any domain. Like the examples discussed in the first Mammon post, AI misalignment stems from unintended consequences rather than malice or rebellion. For example, superbugs don’t emerge because antimicrobials misunderstand or hate us, but because we devised a system that didn’t work the way we assumed it would. The same is true of today's AI systems.[10]10
How reward functions steer optimization
Understanding how reward functions shape AI behavior is key to grasping both the analogy to market capitalism and the nature of optimization itself. At a high level, a reward function is the “carrot and stick” programmers use to motivate an AI to interact with its environment so it learns behaviors that fulfill a goal. Known as reinforcement learning (RL), this reward-maximizing process draws partial inspiration from dopamine-driven learning in animals. As such it somewhat resembles operant conditioning, like training a dog to sit using treats.[11]11
In both machines and organisms, learning can be understood as an optimization process: the goal is to increase the frequency of desired behaviors in response to specific stimuli. In this sense, reward functions (or dopaminergic responses) “steer” behavior by reinforcing particular behavioral patterns over others.
Most designed optimization systems include a steering mechanism. In fact, the prefix in cybernetics comes from the Greek word kybernetes, meaning “steersman.” Steering is what keeps optimization processes adaptive rather than blindly linear.
Profit as a reward signal and steering mechanism in markets
In market capitalism, profit, or money exceeding operating costs, acts as a reward signal[12]12 that motivates people and corporations to participate in the economy. While no explicit “reward function” exists for participants in the economy, the profit motive incentivizes the search for solutions to resource allocation problems. In other words, people pay firms to meet their needs, and that payment incentivizes profitable solutions. In this way, market capitalism can be considered an optimization process–specifically a search algorithm for acceptable solutions driven by firms optimizing for profits. By optimizing for profitability, firms ensure that they’re only investing resources in things people actually want to pay for.
Well, that’s the short version. While this is an elegant and even alluring story, it’s not the whole picture. The biggest wrinkle to the efficacy of market capitalism as a search for solutions to solve distribution problems is information asymmetry. While traditional economics relegates principal-agent problems to special cases, like employment, insurance, and contract law, I would argue that this problem is intrinsic to most aspects of market capitalism.[13]13
“Alignment” between a seller’s offerings and a buyer’s actual wants and needs requires an optimal amount of information symmetry. However, as the complexity of the goods and services offered in the economy increases, most producers will inherently know more about what they’re selling and can misrepresent their product to make excess profits.
The quintessential example is the snake oil salesman, which has become a catch-all for businesspeople with dubious ethics. However, even when a producer provides a product that works, they can very well offer something at a steep but hidden price for the buyer or for third parties not involved in the transaction. Sometimes, this happens because the producer genuinely is unaware of the harms of their product. For example, pollution was poorly understood at the beginning of industrialization, even though it had health and environmental harms. Other times, however, this price is deliberately obfuscated for the producer’s benefit.
For me, a single man is the paradigmatic example of both types of hidden costs and how they illustrate market capitalism’s weak-link problem. Thomas Midgley Jr. (1889-1944)[14]14 was the inventor of tetraethyl lead, or TEL (leaded gasoline), and chlorofluorocarbons (CFCs). These inventions single-handedly created two of the greatest health and environmental disasters of the 20th century.
Profit as a reward signal for deception
Knowledge of lead as a toxin dates back to antiquity; medical documentation describing the deleterious effects of lead exposure dates back as far as the 2nd century BCE.[15]15 By the 20th century, knowledge of lead’s harms wasn’t speculative, although they were poorly quantified. The lead, car, and oil industries would come to operate within this knowledge gap.
Midgley was an early 20th-century General Motors (GM) employee who had been charged with solving the challenge of engine knocking—an issue which reduced the efficiency of the gasoline combustion process—alongside his mentor and boss, Charles Kettering. The pair had tried many combinations of additives and fuels, even patenting a few of these, before settling on TEL as an additive in petroleum gasoline.
Midgley publicly endorsed TEL, once dipping his hands in it and inhaling its vapors, but his private correspondence during his search for a solution paints a more conflicted picture. Very early on, Midgely knew that an ethanol-based additive was viable, having driven a high compression-engine vehicle from Dayton, Ohio, to Indianapolis, Indiana, on a mix of 30% ethanol.[16]16 Later in his research, when he began working with lead, Midgely experienced lead poisoning and had to take a personal holiday to recover.
In the earliest days of car combustion engines, chemists and mechanics more openly experimented with other fuel sources besides gasoline. While engines powered entirely by alcohol were not economically viable, by the 1920s, in most developed nations, people were doing what Midgely did earlier in his research—using gasoline with ethanol to prevent engine knocking.
If Midgely and other chemists knew ethanol worked as an additive, what changed? In 1920, when DuPont became a majority stakeholder of GM, the concern went from solving the engine-knocking problem for its own sake to cornering the fuel market with an exclusive anti-knocking agent that would be essential to the future of gasoline. What’s more, Kettering and Midgley had very little time to deliver this solution. TEL served this role dutifully. It was patentable and would allow GM to earn profits from most of the gasoline sold in the US until leaded gasoline was phased out in 1996.
Oddly, even after TEL’s approval by DuPont in 1921, Kettering’s lab continued researching ethyl alcohol and the possibility of using alcohol-based fuels. This research, driven by Midgely, seems to have come from a genuine desire to make the US less reliant on foreign oil via a renewable fuel source. Regardless of what Midgely wanted, further investigation was ultimately quashed in 1923. DuPont wasn’t interested in any of this research, as they couldn’t patent other chemical blends. Furthermore, GM’s burgeoning relationship with Standard Oil of New Jersey (today, ExxonMobil) shifted the organization’s view on the viability of non-petroleum based fuels for reasons that should be obvious.
Almost immediately, TEL’s adoption faced controversy forcing DuPont, GM, and Standard Oil to manage growing concerns over the chemical’s safety. Within months of the initial production of TEL, workers producing the substance fell ill, went insane, and some eventually died. Several public health experts came out against TEL, fully aware of the harmful effects of lead, with some states in the US (like New York) initially banning it. Ultimately, this brief clash over public safety was quickly abandoned and largely forgotten. For their part, Midgley and Kettering went on to be hailed as rock stars of innovation, with their legacy only recently having come into doubt.
The societal cost of leaded gasoline cannot be understated, and we’re still learning about its harms and impacts. But from what we’ve learned, these are staggering. TEL caused millions of premature deaths and a substantial increase in cognitive developmental issues, as well as lifelong mental health problems among hundreds of millions of people across the world for the past century. Some estimates suggest that eliminating leaded gasoline has generated $2.4 trillion in annual benefits globally. This is arguably the largest “subsidy” that a company has enjoyed at the expense of the public. This is especially damning given that safer alternatives existed all along—just not ones companies could easily profit from. The kicker is that the switch from leaded to unleaded gasoline occurred as a byproduct of emission regulations not explicitly targeting lead as an additive.
Profit as a reward signal for increasing information asymmetry to capture regulation
Midgley, GM, and DuPont would go on to proceed over a second 20th-century environmental catastrophe—the depletion of the Earth’s natural ozone. Midgely, again playing the role of disastrous inventor, created a CFC known as Freon. It was a refrigerant replacing older, more dangerous refrigerants in use at the time, like ammonia. Freon was safe to humans in the quantities used in industry, so in this case, Midgely genuinely couldn’t anticipate its consequences. It wasn’t until the 1980s, when the technology to extensively monitor the upper atmosphere was developed, that the problem became apparent. Soon after, these chemicals would be banned after the ratification of a global treaty called the Montreal Protocol.
Though the 1987 Montreal Protocol is often hailed as a climate success, it followed a familiar corporate playbook, as described in Merchants of Doubt by Naomi Oreskes and Erik M. Conway. Satellite imaging provided overwhelming evidence of the ozone hole and scientists knew about the CFC issue as early as the 1970s. Like with leaded gasoline, the scope of the harms here were huge, although the issue was caught relatively early before the worst of these harms could be realized. Should the hole have been allowed to grow further, it would have increased incidents of cancer, broken down ecosystems by killing organisms and plants, and created force-multiplying effects for climate change.[17]17
From the outset, DuPont sought to shape scientific narratives, sow uncertainty, and delay regulation. It wasn’t until the company built inroads with the Reagan Administration that its strategy shifted from stalling regulation to participating in negotiations. As CFC profits declined, DuPont leveraged the negotiations to fund and dominate the search for alternatives. By the mid-1980s, DuPont was on board with regulations, not because of the overwhelming force of the science but because it had put itself in a position to lead the discussion on the ozone layer issue. In essence, the Montreal Protocol was a way to gain a competitive advantage over other companies in the same industry. DuPont even resisted congressional and public pleas to stop producing CFCs until 1995, the final phase-out date specified in the Montreal Protocol.
While the success of the Montreal Protocol shouldn’t be understated, one of its most important lessons is that negative externalities only get fixed when a large conglomerate’s economic interests happen to align with the public’s. Even then, at every phase of DuPont’s approach to the CFC issue, it was able to shape the perception of the science. By the time the ozone hole was documented, DuPont appeared to be a proactive, thoughtful company working to improve the industry, as opposed to the reluctant partner it had been just a decade prior. In some counterfactual world, where the economics of CFCs remained more viable, it seems plausible that DuPont very well may have behaved as the oil industry is today on climate change.
While Mammon is the god of unintended consequences, lurking at the heart of many of our most wicked problems, it’s essential to draw attention to our core problem-solving system, market capitalism. In future posts we'll analyze capitalism to better understand the mix of incentives it creates for firms.
Summary
- AI systems and the methods used to train them can be understood as forms of optimization, meaning they're subject to optimization failures highlighted in earlier posts.
- AI alignment is the effort to ensure these systems optimize for the right outcomes, avoiding unintended or harmful behavior.
- Because AI systems are generally designed to take actions on behalf of users there's a strong analogy between AI alignment failures and market failures.
- Like with other optimization processes, it’s very easy to misspecify an AI systems objectives when building a reward function, such that it “mis-optimizes” or does the wrong thing.
- In market capitalism, profit can be thought of as a reward signal that motivates economic actors to solve a problem. However, it can also incentivize deceptive or harmful behavior if those actions are profitable.
- Ideally, firms get paid only when they deliver real value. But information asymmetry allows companies to profit from suboptimal or even dangerous goods and services. This is increasingly more common as the technical complexity of goods and services in the economy requires domain expertise to fully understand: industrial chemicals, artificial intelligence, pharmaceuticals, etc.
- As a result, market capitalism becomes a “weak-link” system, where the worst actors disproportionately undermine the whole system. This mirrors fears in AI alignment: it only takes a few misaligned agents to create catastrophic risks.
Recommended resources
- Book: The Alignment Problem: Machine Learning and Human Values by Brian Christian
- Paper: Consequences of Misaligned AI by Simon Zhuang and Dylan Hadfield-Menell[18]18
- Book: Merchants of Doubt: How a Handful of Scientists Obscured the Truth on Issues from Tobacco Smoke to Climate Change by Naomi Oreskes and Erik M. Conway
- Book: Phishing for Phools: The Economics of Manipulation and Deception by George A. Akerlof and Robert J. Shiller
- Book: The Moral Economy: Why Good Incentives Are No Substitute for Good Citizens by Samuel Bowles
- Dark PR: How Corporate Disinformation Harms Our Health and the Environment by Grant Ennis
- Book: Risk Society: Towards a New Modernity by Ulrich Beck
- Book: Unleaded: How Changing Our Gasoline Changed Everything by Carrie Nielsen
- Book: Deceit and Denial: The Deadly Politics of Industrial Pollution by Gerald Markowitz and David Rosner
- Dissertation: The Ethyl Controversy: How the news media set the agenda for a public health controversy over leaded gasoline, 1924-1926 by Bill Kovarik[19]19
- Video: Leaded Gasoline Environmental History Centennial Panel including Dr. Howard Mielke, Dr. Caroline Nielsen, Dr. Jerome Nriagu, Dr. David Rosner, Dr. David Egilman, Dr. Bill Kovarik, and Mr. Thomas Beller
Affiliate disclaimer
Books on this page and throughout the site link to my Bookshop.org affiliate pages, where your purchase will earn me a commission that will go towards covering site costs. If you at all find the books I recommend interesting, consider purchasing through Bookshop, which shares profits with local bookstores. You can learn more about how you can support Misaligned Markets here.
- The Turing Test has always been an informal standard applied to AI performance, but nonetheless it shapes people's perceptions of intelligence and is still an area of academic inquiry.
- Colloquially some divide AI safety literature into "alignment" and "ethics." Increasingly some non-alignment AI and technology researchers have pushed against framing AI safety around superintelligence. See: Lee Vinsel on CritHype, Arvind Narayanan and Sayash Kapoor on Normal AI, Emily Bender on Stochastic Parrots, and Timnit Gebru and Émile Torres on TESCREAL.
- See Sam Bankman-Fried, the temporary ouster of Sam Altman from OpenAI, and the Zizians.
- Math, design, and engineering students often encounter concepts like control theory or cybernetics, but rarely study them as standalone fields.
- The role of optimization in AI is pretty well understood by people developing AI, many of whom only have a passing interest in “AI alignment.” Optimization in this case can be thought of as a mathematical way of finding the proper path for an AI to take given the “shape” of the data it has encountered.
- As far as I know, Christian has no direct connection to the online rationalists or academic AI alignment researchers and seems to have taken up interest in this topic through personal conversations with Peter Eckersley (also mentioned in this post).
- Christian's book is interesting in that he's a technical outsider who takes a very broad view towards alignment which includes considerations from both the alignment and ethics "camps" in the AI safety field.
- Machine learning (ML) and deep learning (DL) are techniques for building AI systems, though conceptually, ML and DL are the same. Agentic AI refers to multimodal AI capable of performing tasks like coding or searching the internet independently. For instance, Gemini 2.5 is an AI model that can process video, audio, and text within a single conversation or over API.
- Specification gaming is analogous to the idiom of “gaming the system.” A model will perform an action that satisfies the criteria of its reward function but not actually engage in helpful behavior. This can also emerge when the reward function only works in a training environment that does not resemble a deployed environment.
- As AI developers rush to release poorly calibrated autonomous systems some fears articulated in AI alignment literature will likely be realized. This is true even if we never reach superintelligence. For me, an anecdote that brought this home involved a LangChain AI agent using a CLI to randomly deploy software packages on a user's computer.
- This is a huge simplification, admittedly. In many cases, reinforcement learning (RL) involves training a population of agents rather than a single agent, so the parallel to operant conditioning is not completely realistic.
- I switched from using the term reward function to "reward signal" when talking about economies because people and firms don’t have a “reward function” in a literal sense (although something like utility theory might try to approximate one).
- George Akerlof’s “Market for Lemons” is a seminal paper that analyzes information asymmetry in the context of car markets. This is treated as an edge case in introductory economics courses. I argue that as economies become more complex many markets in capitalist systems are altered by information asymmetry. See corponomics/corporate kung fu. Future blog posts will elaborate on the flow of information in capitalism.
- It’s an inside joke among my friends that I’m obsessed with this man. Feel free to join in the drinking game whenever I say his name in future posts. As someone whose decision harmed and disabled tens of millions of people over a century, Midgley’s a perfect example of the upper bounds of harm that one person can have following market incentives.
- Specifically, lead is a neurotoxin that impairs adult brain function. In children, it prevents the development of certain parts of the brain, which impacts reasoning, impulse control, and other critical aspects of brain health. Children exposed to lead are more likely to have cognitive or developmental disorders; however, knowledge of issues in children wasn’t well known until the 20th-century.
- Today, E30 is a common blend of unleaded gasoline.
- The ozone hole still exists, and the Montreal Protocol does not prohibit every known chemical that depletes ozone, but what we’ve done is buy time for it to replenish naturally by banning the most significant contributors to ozone breakdown. This is undoubtedly a success, but it’s important to stress that this issue has not been fully resolved and must be managed by governance. It is a progress trap.
- Math alert: For a somewhat less technical overview on a related topic, see this video from Dylan Hadfield-Menell (also linked in the blog).
- Much of what we know about DuPont, GM, and Midgely on the leaded gasoline controversy comes from the research of Bill Kovarik, who I've linked to liberally throughout this post. Even links that aren't to his site reference his research. I encourage you to engage with his work directly.